안녕하세요 오늘은 CycleGAN의 Generator와 Discriminator를 pytorch로 구현해보았습니다.
CycleGAN의 Generator는 U-Net 구조나 ResNet 구조를 이용하는데
저는 Resnet 구조로 구현 해보았습니다. (ResNet block 9개)
참고 페이지 : https://github.com/junyanz/pytorch-CycleGAN-and-pix2pix/tree/003efc4c8819de47ff11b5a0af7ba09aee7f5fc1
GitHub - junyanz/pytorch-CycleGAN-and-pix2pix: Image-to-Image Translation in PyTorch
Image-to-Image Translation in PyTorch. Contribute to junyanz/pytorch-CycleGAN-and-pix2pix development by creating an account on GitHub.
github.com
Generator (ResNet)
※ Convolution 연산 전 'reflect padding'을 더하여 Feature map의 size를 유지시키는 것 같습니다.
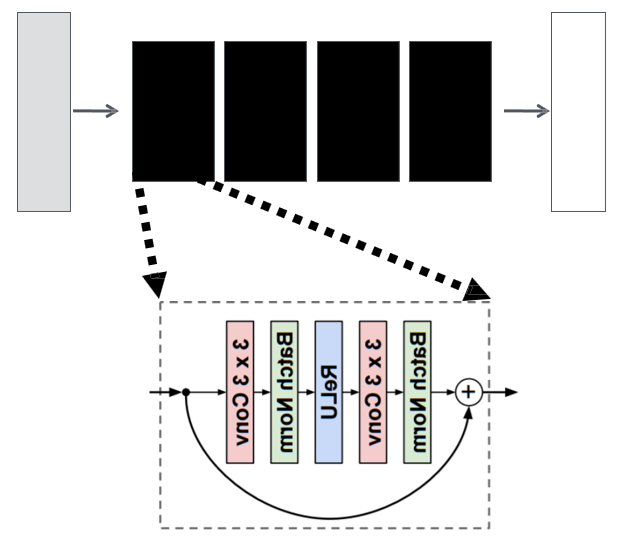
#CycleGAN Generator(ResNet)
class ResnetBlock(nn.Module):
def __init__(self, dim, padding_type, norm_layer, use_dropout):
super(ResnetBlock, self).__init__()
self.conv_block = self.build_conv_block(dim, padding_type, norm_layer, use_dropout)
def build_conv_block(self, dim, padding_type, norm_layer, use_dropout):
conv_block = []
p = 0
if padding_type == 'reflect':
conv_block += [nn.ReflectionPad2d(1)]
else:
p = 1
conv_block += [nn.Conv2d(dim, dim, kernel_size=3, padding=p)]
if norm_layer == 'BatchNorm':
conv_block += [nn.BatchNorm2d(dim)]
elif norm_layer == 'InstanceNorm':
conv_block += [nn.InstanceNorm2d(dim)]
conv_block += [nn.ReLU()]
if use_dropout:
conv_block += [nn.Dropout(0.5)]
p = 0
if padding_type == 'reflect':
conv_block += [nn.ReflectionPad2d(1)]
else:
p = 1
conv_block += [nn.Conv2d(dim, dim, kernel_size=3, padding=p)]
if norm_layer == 'BatchNorm':
conv_block += [nn.BatchNorm2d(dim)]
elif norm_layer == 'InstanceNorm':
conv_block += [nn.InstanceNorm2d(dim)]
return nn.Sequential(*conv_block)
def forward(self, x):
out = x + self.conv_block(x)
return out
class Generator(nn.Module):
def __init__(self):
super().__init__()
model = []
# input image => RGB 3 channel
model += [nn.ReflectionPad2d(3),
nn.Conv2d(3, 64, kernel_size=7, padding=0),
nn.InstanceNorm2d(64),
nn.ReLU()]
# downsampling x 2
model += [nn.Conv2d(64, 128, kernel_size=3, stride=2, padding=1),
nn.InstanceNorm2d(128),
nn.ReLU()]
model += [nn.Conv2d(128, 256, kernel_size=3, stride=2, padding=1),
nn.InstanceNorm2d(256),
nn.ReLU()]
# Residual block x 9
n_blocks = 9
for i in range(n_blocks):
model += [ResnetBlock(dim=256, padding_type='reflect', norm_layer='InstanceNorm', use_dropout=False)]
# Upsampling x 2
model += [nn.ConvTranspose2d(256, 128, kernel_size=3, stride=2, padding=1, output_padding=1),
nn.InstanceNorm2d(128),
nn.ReLU()]
model += [nn.ConvTranspose2d(128, 64, kernel_size=3, stride=2, padding=1, output_padding=1),
nn.InstanceNorm2d(64),
nn.ReLU()]
# output
model += [nn.ReflectionPad2d(3),
nn.Conv2d(64, 3, kernel_size=7, padding=0),
nn.Tanh()]
self.model = nn.Sequential(*model)
def forward(self, x):
return self.model(x)
학습을 진행하지 않은 상태로 Generator 모델에 이미지를 넣어보았는데 아래와 같은 결과가 나왔습니다.
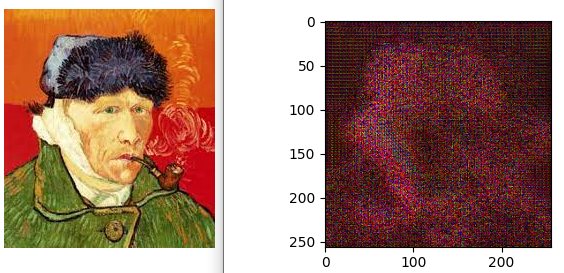
Discriminator (70x70 Patch GANs)
CycleGAN 논문 내용중에 Discriminator 모델은 '70x 70 Patch GANs' 모델을 사용하였다고 나와있습니다.

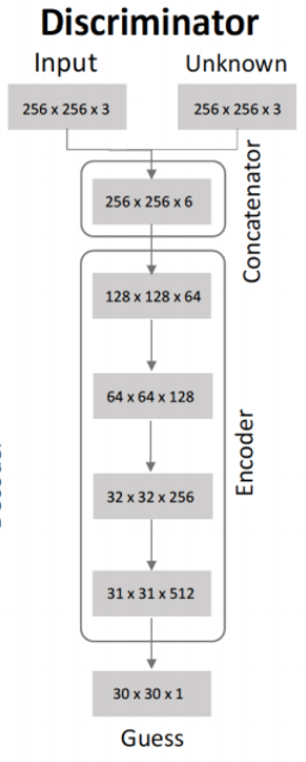
'70 x 70 Patch GANs' 의 모델 구조를 찾아보았고 아래와 같이 구현하였습니다.
# Discriminator => PatchGANs 70x70
class Discriminator(nn.Module):
def __init__(self):
super().__init__()
model = []
model += [nn.Conv2d(6, 64, kernel_size=4, stride=2, padding=1),
nn.LeakyReLU(0.2)]
model += [nn.Conv2d(64, 128, kernel_size=4, stride=2, padding=1),
nn.BatchNorm2d(128),
nn.LeakyReLU(0.2)]
model += [nn.Conv2d(128, 256, kernel_size=4, stride=2, padding=1),
nn.BatchNorm2d(256),
nn.LeakyReLU(0.2)]
model += [nn.Conv2d(256, 512, kernel_size=4, stride=1, padding=1),
nn.BatchNorm2d(512),
nn.LeakyReLU(0.2)]
model += [nn.Conv2d(512, 1, kernel_size=4, stride=1, padding=1),
nn.Sigmoid()]
self.model = nn.Sequential(*model)
def forward(self, x):
return self.model(x)
학습을 진행하지 않은 상태로 Discriminator 모델에 이미지를 넣어보았는데 아래와 같은 결과가 나왔습니다.
Discriminator 모델의 output은 30 x 30 입니다.
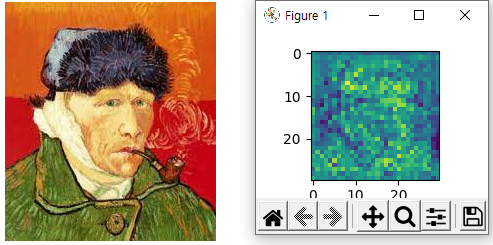
다음으로 각 모델을 학습 시킬수 있도록 Loss function 도 구현 해보고
학습을 시켜본 후 포스팅 하도록 하겠습니다.
'AI' 카테고리의 다른 글
(U-Net) pytorch로 Mirroring Extrapolate를 적용하여 학습하기 (0) | 2022.05.18 |
---|---|
(U-Net) pytorch로 Mirroring Extrapolate 구현하기 (0) | 2022.04.13 |
(U-Net) 모델 분석 및 Pytorch로 구현 - 1 (2) | 2022.04.12 |
(Pytorch) Linear Regression, Multiple Linear Regression (0) | 2022.04.06 |